Abstract
While initial beam alignment (BA) in millimeter-wave networks has been thoroughly investigated, most research assumes a simplified terminal model based on uniform linear/planar arrays with isotropic antennas. Devices with non-isotropic antenna elements need multiple panels to provide good spherical coverage, and exhaustive search over all beams of all the panels leads to unacceptable overhead. This paper proposes a location- and orientation-aware solution that manages the initial BA for multi-panel devices. We present three different neural network structures that provide efficient BA with a wide range of training dataset sizes, complexity, and feedback message sizes. Our proposed methods outperform the generalized inverse fingerprinting and hierarchical panel-beam selection methods for two considered edge and edge-face antenna placement designs.
Figures
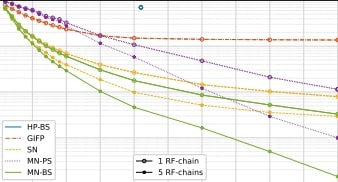
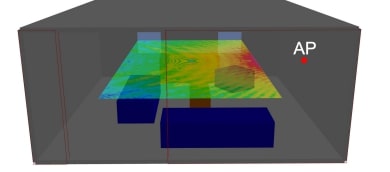
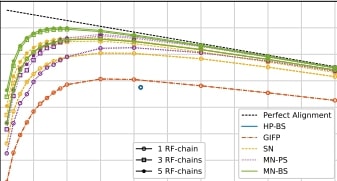
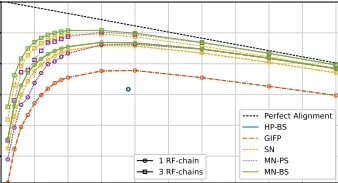
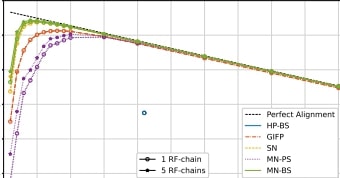
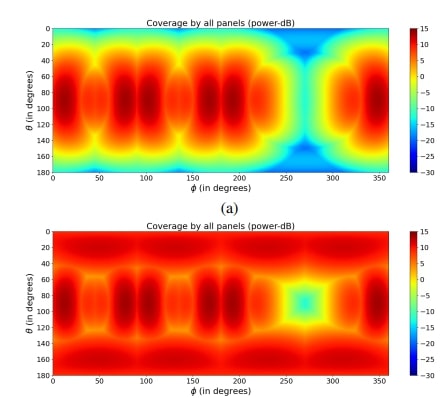
Bibtex
@INPROCEEDINGS{10001089,
author={Rezaie, Sajad and Morais, João and de Carvalho, Elisabeth and Alkhateeb, Ahmed and Manchón, Carles Navarro},
booktitle={GLOBECOM 2022 - 2022 IEEE Global Communications Conference},
title={Location- and Orientation-aware Millimeter Wave Beam Selection for Multi -Panel Antenna Devices},
year={2022},
volume={},
number={},
pages={597-602},
keywords={Training;Performance evaluation;Learning systems;Millimeter wave devices;Neural networks;Turning;Linear antenna arrays;millimeter wave;beam alignment;location-aware;orientation-aware;multi-panel},
doi={10.1109/GLOBECOM48099.2022.10001089}}